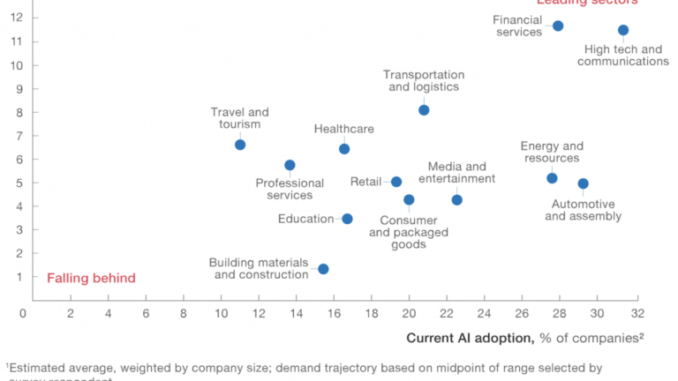
30-second summary:
The potential of artificial intelligence is almost boundless. AI solutions are starting to be introduced by organizations across many industries and fields.
A strong starting point for any AI implementation is to get buy-in from company decision-makers. If key stakeholders understand AI’s potential, they’ll properly resource any transition.
AI is particularly useful in the field of data analytics. If you are going to join the ‘Big Data’ era, you need robust data governance.
There’s no one-size-fits-all approach to AI implementation. You need to explore and test the different tools and solutions available to you.
Don’t neglect the human side of your business in seeking AI-driven answers to your problems. Make sure you train staff effectively in all automation or AI tools you introduce.
It’s not insider information to know the unmatched potential of company-wide AI implementation. Even with all the advancement in recent years, it still feels like we’re only beginning to see what artificial intelligence can do.
There are countless examples of enterprises across dozens of sectors using AI for diverse tasks and processes. Algorithms help firms to predict customer behavior and buying patterns, optimize supply chains, personalize experiences, understand your workforce, and even help you find Waldo.
For some companies, though, implementing and accelerating full-scale implementation is a daunting prospect. Many have concerns over vendors, integration ability, cost, and privacy and regulatory issues. Is the juice even worth the squeeze given these challenges?
So, if you’re thinking of further adopting AI into your processes, or you have begun the transition and are finding it frustrating or tedious, here are five ways to reach your goals quicker.
Source: McKinsey & Company
1) Secure executive sponsorship
Like SaaS examples before it, AI is ushering in a new way to do things compared to on-premise software. But with the change, comes challenge. Having C-suite buy-in is crucial for success.
The more informed and engaged the higher-ups are in the uses of AI, the better the chances of enterprise-wide adoption. “Strong executive leadership goes hand-in-hand with stronger AI adoption.
Respondents from firms that have successfully deployed an AI technology at scale tended to rate C-suite support nearly twice as high as those from companies that had not adopted AI technology,” according to this McKinsey Global Institute study.
If there’s no business leader positioned to take the lead of your AI transition, you’re already off to a bad start. Make sure that those in executive positions are tasked with different facets of an AI integration program.
Each step also must be staffed appropriately to drive the process, without being afraid to change the management over the course of a campaign to be successful.
Schedule a weekly teleconference with the key stakeholders to ensure roles are constantly refined, and everyone’s kept in the loop in terms of the adoption status.
It’s also worth stressing that you – as the head of this campaign – need to be able to dictate resources, investment, and overall strategy across the organization. This includes actively engaging those around you for support with AI strategy, human and IT assets, and cultural adoption.
It would help if you made cultural adoption a priority by holding organizational leaders accountable as they execute the revisions needed to continue the transformation. C-suite must remove barriers and obstacles, both technical and cultural, to increase your chances of success.
Once C-suite is aligned with your goals, you need to determine how you wish to manage and control the budget. That’s especially true if your current landscape is made up of competing internal analytics or AI efforts.
Lastly, don’t forget to celebrate and communicate progress to your organization. This helps bolster the commitment from executives as well as gain support for the transformation.
Source: McKinsey & Company
2) Define data management and governance
Smarter and more accessible ‘self-service’ and team collaboration software brings with it an increase in data, data sources, and more end-user expectation.
As a result, the demand for proper data governance becomes essential. Without it, the data sits without a purpose in a data lake or warehouse. Look at it this way, more data without restriction can give businesses more freedom.
However, at an enterprise level, it can mean missed steps, inefficient outputs, and oversights. Faster analytics may become a problem before it feels like a solution.
It’s critical to address this with support from executives. This means defined resources to manage and enhance data collection, efficiency, and usage across all vital functions.
The data governance team must, additionally, set out and oversee data policies, standards, definitions, and manage data quality.
Remember, not all data is equal. Define what needs executive control, and which data can be made publicly available for use.
Given today’s availability of more user-friendly analytics and visualization tools, how much ‘self-service’ can be allowed to create better predictive models or different ways of creating new business processes? Who can define these datasets and use cases?
These are vital aspects to consider, as there’s a balance that needs to be struck between being rigid and protective and being flexible. This, again, highlights the importance of a useful data governance model.
Too much control can mean slow processes, lack of response, red-tape, the need for things like email verification, and overt use of business-led IT solutions.
Too much flexibility can mean different versions of the truth, leading to no real ownership or responsibility, conflict, and a reduction in productivity.
Source: KPMG
As you make decisions about AI, a data governance process allows you to implement and manage said decisions. Including who can access what, how much access, and what that access entails.
3) Take a consider and test approach as opposed to success or failure
All AI adoptions are unique and present their own sets of challenges. And so, you need to begin all AI introductions with a ‘test and refine’ method as opposed to a ‘success or failure’ approach.
Conventionally, analytical methods infer a defined relationship between variables. Trialing a one-sided hypothesis will either validate or reject it, but won’t uncover the hidden connection between the variables; the why.
Creating hypotheticals for each step, and then using these learnings and experiences through the next ones is critical. It means refining and curating your AI deployment until it feels like a workable solution that delivers meaningful results is a much easier process.
And, while this approach will inevitably extend deployment deadlines, it also allows you to fine-tune the outcome to incorporate real-life lessons learned.
If you’re integrating AI into computerised customer service like automated chatbots, it’s vital no matter where the customer goes there’s an answer waiting for them. It can’t work up until a certain point, it needs absolutes. Ultimate solutions will then align with the employee and end-user needs.
Source: PWC
4) Spend time on change management and training
Deploying an AI API to ingest a new dataset is straightforward. However, altering the management and training for analysts who’ll be using these processes going forward is a challenge.
Most forms of AI create automated decisions – “yes” or “no.” However, it is often the case that the integration of ML algorithms can allow for more subtle responses as well. These responses may be used in conjunction with existing processes to deliver the best results.
For example, if an AI decision scores say, a loan application on a 1-10 scale of suitability, scores from 7-10 may yield an automatic yes.
However, anything lower will still require human input to grant or deny the application. If you’re integrating AI to analyze voice commands in a call center over VoIP communications, how can it distinguish commands deeper than just “option 1 or option 2”?
Just as you would spend time training employees on how to use a specific process, the same is true for AI-based outcomes.
Human employees may need to spend a few weeks analyzing the results coming back from the AI algorithms. That would give them a frame of reference in terms of how to interpret the scores best.
If you’re using an AI vendor, they can guide in terms of how to understand results and how employees can get the most out of the new system. Otherwise, learning how to create an online learning platform could be a worthwhile investment to get team members up to speed.
AI isn’t ‘magic.’ It’s just a way to understand patterns and behaviors to deliver more accurate results and make predictions. AI only works when it has a defined problem to solve and the right metrics to succeed. If you haven’t clearly defined the issue you’ve bought AI in to solve, you won’t get the right solution.
Source: Harvard Business Review
5) Consolidate and assimilate automation
As you ramp up enterprise-wide AI adoption, what these processes look like in the future will change with the introduction of a multitude of types of automation. From complete manual processes all the way to the adoption of RPA, and even more advanced AI protocols.
It’s best to just (and I know it’s a big just) re-invent business processes from the ground up with AI in mind. You can then apply the best tool for the job at any given step.
Merely inserting RPA or AI into established processes may mean you miss out on all of its potential. You also need to consider the handoffs that need to happen as you further integrate.
This includes human-machine or machine-machine learning. By streamlining the handoffs and making them more seamless and reliable, you can further enhance your future processes to be cost-effective, competitive, and agile.
Source: Harvard Business Review
AI implementation can be sped up. However, it’s not necessarily about being smarter; it’s about making the right choices. Having executive buy-in combined with a defined data governance team is vital.
As is becoming fixated with data quality, dedicating enough time to change management, and having a test without defined expectations approach.
If you’re finding your AI project taking up too much time, be patient. Like any kind of digital transformation, just as you’re approaching the finish line, you’ll likely encounter another hurdle. Overcome it, though, and the possibilities are boundless.
John Allen is the director of global SEO at RingCentral, a global UCaaS, VoIP and video conferencing solutions provider. He has over 14 years of experience and an extensive background in building and optimizing digital marketing programs. He has written for websites such as Hubspot and BambooHR.
The post 5 tips to accelerate your company’s AI implementation appeared first on ClickZ.
Source: ClickZ
Link: 5 tips to accelerate your company’s AI implementation
Leave a Reply